ISO 42001:2023 Annex A. Control 4.3
Explaining ISO 42001 (Annex A. Annex B.) Control 4.3: Data resources
ISO 42001 Annex A Control 4.3, titled "Data Resources," focuses on the systematic documentation of data used in AI systems. This control ensures that your organization identifies and maintains detailed records of data resources to promote transparency, mitigate risks, and uphold the integrity of AI operations.
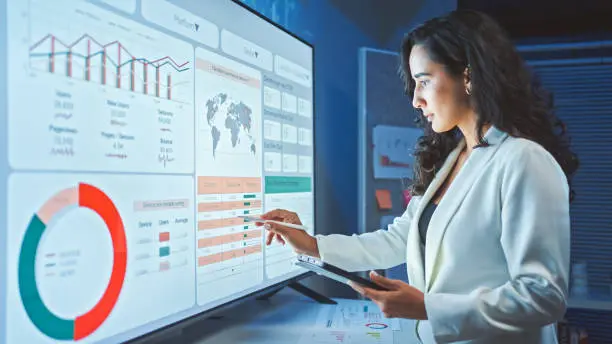
Annex A.4
- Resources for AI systems
Annex B.4
- Resources for AI systems
Annex A.4.1 Objective
- To ensure that the organization accounts for the resources (including AI system components and assets) of the AI system in order to fully understand and address risks and impacts.
Annex B.4.1 Objective
- To ensure that the organization accounts for the resources (including AI system components and assets) of the AI system in order to fully understand and address risks and impacts.
Control A.4.3 Data resources
- As part of resource identification, the organization shall document Information about the data resources utilizedfor the AI system.
Objective of Control 4.3
The primary objective of ISO 42001 Control 4.3 is to ensure that your organization identifies, categorizes, and documents all data resources associated with its AI systems. Comprehensive documentation of data ensures traceability, facilitates risk mitigation, and supports ethical AI development. This control helps organizations establish a reliable foundation for AI systems, ensuring that data used is accurate, relevant, and free from undue bias.
Purpose of Control 4.3
Resource documentation is essential for your organization to:
- Manage Risks: It helps identify gaps or issues in resource allocation, reducing potential operational disruptions.
- Enhance Transparency: Clear documentation enables all stakeholders to understand the resources used in AI system development and operation.
- Support Audits and Assessments: Comprehensive records are critical for internal audits and external compliance reviews.
- Optimize Resource Utilization: Knowing what resources are available can help in planning future AI projects efficiently.
Introduction to Data Resource Documentation
Data is the main source of AI systems, and the quality of these systems directly depends on the reliability of the data. Documenting data resources is therefor one of the most important things to ensure that the AI system operates as intended and produces trustworthy results. For your organization, this documentation serves as a reference point for understanding how data is sourced, processed, and utilized throughout the AI lifecycle. Proper documentation minimizes risks, improves decision-making.
Elements of B.4.3 Data Documentation
1. Data Provenance
Provenance refers to the origin and history of data. Your organization must document:
- The source of the data (e.g., collected internally or from third parties).
- Methods used for data collection.
- Any transformations or preprocessing steps applied to the data. Understanding the provenance ensures that data is credible and aligns with the intended purpose of the AI system.
2. Data Update and Modification History
Maintaining records of data updates is vital for ensuring its relevance and accuracy. Documentation should include:
- The date and time of the last update.
- Specific changes made to the data.
- Metadata reflecting these updates. This helps your organization track the currency of data used in AI systems.
3. Data Categorization
Categorization is essential for organizing data and ensuring its appropriate use in AI workflows. Your organization should classify data into categories such as:
- Training data: Used for developing AI models.
- Validation data: Used for fine-tuning models.
- Test data: Used for evaluating model performance.
- Production data: Data used in real-world applications. Referencing ISO/IEC 19944-1 provides a standardized approach to data classification.
4. Data Labeling Processes
The accuracy of labeled data determines the effectiveness of machine learning models. Your organization should document:
- Procedures for labeling data.
- Tools and techniques used for labeling.
- Measures to ensure consistency and accuracy. Improperly labeled data can lead to incorrect AI predictions, so robust labeling processes are critical.
5. Intended Use of Data
Each dataset should have a clearly defined purpose. Your organization must document the intended use of the data to:
- Align with AI system objectives.
- Prevent misuse of data.
- Support transparency in AI operations.
6. Data Quality Assurance
Data quality has a significant impact on AI system outcomes. To ensure high-quality data, your organization should:
- Establish data quality criteria (e.g., accuracy, completeness, consistency).
- Regularly assess data against these criteria.
- Reference standards like the ISO/IEC 5259 series for guidance. Documenting quality assurance measures ensures that data used in AI systems is reliable and fit for purpose.
7. Data Retention and Disposal Policies
Your organization must have clear policies for data retention and disposal, including:
- Retention schedules based on legal and operational requirements.
- Secure methods for disposing of obsolete or redundant data. Adhering to these policies prevents unauthorized access to sensitive data and supports compliance.
8. Identifying and Mitigating Data Bias
Bias in data can skew AI outcomes, leading to unfair or inaccurate results. To address this, your organization should:
- Document known or potential biases in datasets.
- Implement strategies to identify and mitigate these biases.
- Regularly audit data for representativeness and fairness. Transparent documentation of bias mitigation efforts enhances the trustworthiness of AI systems.
9. Data Preparation Techniques
Data preparation is a critical step in AI system development. Your organization should document processes such as:
- Data cleaning: Removing errors or inconsistencies.
- Data transformation: Structuring data for analysis.
- Data augmentation: Enhancing datasets with additional information. These steps ensure that data is ready for effective use in AI workflows.
Compliance and Ethical Considerations
Adhering to ISO 42001 Control 4.3 demonstrates your organization’s commitment to compliance and ethical practices. Documentation of data resources supports legal compliance, facilitates audits, and upholds ethical standards in AI operations.
Tools and Technologies for Data Documentation
Modern tools can simplify the process of data documentation. Your organization can use:
- Data cataloging software to track and manage datasets.
- Metadata management tools to document data characteristics.
- AI-specific platforms that integrate documentation features within workflows.
Challenges and Best Practices
Common Challenges
- Managing large volumes of data.
- Ensuring consistency in documentation across teams.
- Identifying and mitigating biases in complex datasets.
Best Practices
- Develop standardized templates for data documentation.
- Train staff on documentation requirements and practices.
- Perform regular audits to verify documentation accuracy and completeness.