Detailed Breakdown of ISO 42001 Annex D
Annex D of ISO/IEC 42001 is an informative annex that emphasizes the broad applicability of an AI Management System (AIMS) across industries and sectors. It underscores that the standard’s framework for trustworthy and responsible AI is universally relevant to organizations in diverse domains, whether they develop, provide, or use AI systems.
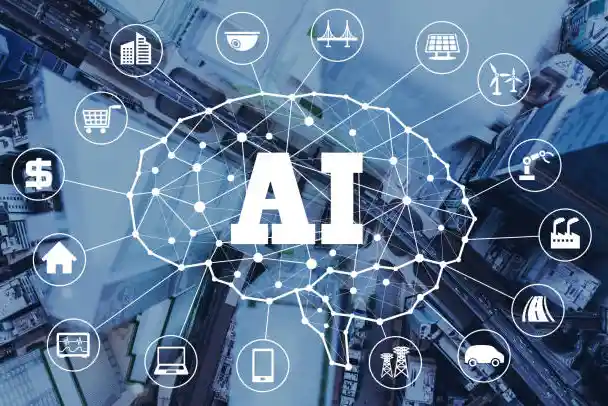
Annex D. Use of the AI management system across domains or sectors
The annex highlights “AI-specific considerations” (e.g. dealing with opaque algorithms or continuous learning systems) and the broader ecosystem of technologies that feed into AI, underscoring a holistic approach to responsible AI development and use.
AI Management Across Domains (Annex D)
Importantly, Annex D advocates integrating the AI management system with other generic or sector-specific management system standards as part of this broad applicability. By doing so, organizations ensure that AI-related risks are managed in conjunction with other operational risks and industry best practices. Annex D provides general guidance for applying ISO 42001 in specific industries – for example, it explicitly mentions healthcare, finance, and defense as sectors where the AIMS can be applied, and stresses the importance of aligning AI management with any existing sector-specific standards or regulations. This general guidance helps organizations accomodate the AIMS to their context, acknowledging that each industry faces unique challenges (e.g. patient safety in healthcare, or mission-critical reliability in defense). Annex D ensures that AI governance is context-aware and that the AI management system remains effective and compliant in any domain.
Annex D is significant because it reinforces that ISO 42001’s principles are broadly applicable – AI trustworthiness and risk management are relevant whether you’re running a bank, a hospital, a manufacturing plant, or a government agency. It encourages organizations to embed AI management into their existing management frameworks and to be mindful of industry-specific requirements and scenarios when implementing their AI governance. This annex fundamentally acts as a bridge between the universal requirements of the AI management system and the specialized needs of various sectors, ensuring the standard can be adopted flexibly yet consistently across the board.
Integration with Other Management System Standards
One of the strengths of ISO 42001 is that it shares a common high-level structure with other ISO management system standards, making integration more straightforward. Annex D (and the related Appendix D in the standard) provides guidance on how an AIMS can be combined with or incorporated into existing management systems.
Because ISO 42001 follows the same ISO “High-Level Structure” (HLS) as standards like ISO 27001 and ISO 9001 – with identical clause numbers for Context, Leadership, Planning, etc. – organizations can align and merge these systems more easily. This harmonized structure reduces duplication of effort and facilitates a unified governance framework.
Next we discuss how an AI management system can integrate with key standards in security, privacy, quality, and sector-specific domains, highlighting similarities, differences, and alignment strategies:
ISO/IEC 27001 (Information Security Management)
ISO 27001 provides a framework for managing information security risks (protecting confidentiality, integrity, availability of information). ISO 42001 and ISO 27001 are highly complementary and were “designed to be combined” under a unified management system. Both require risk assessment, access control, incident response, continuous improvement, etc., so many of their clauses and controls overlap.
With integrating an AIMS with an existing ISMS, organizations can harmonize policies and procedures to ensure that protecting sensitive data and managing AI risks go hand-in-hand. For example, AI systems often process sensitive data, so the AIMS must enforce data security and privacy controls just as the ISMS does. Aligning these systems means an organization can have one consolidated risk management process covering both AI and information assets. In practice, integration involves mapping the AI management requirements to existing security controls – for instance, extending the scope of an incident response plan to include AI system incidents, or updating supplier security requirements to address AI components.
The benefit is a consistent, enterprise-wide approach to governance: employees get a unified set of training and awareness programs (covering both AI ethics and infosec) and management receives integrated reports and audits for both domains. Organizations already certified to ISO 27001 find it efficient to add ISO 42001, since the structures and objectives align closely, and they mainly need to implement the additional AI-specific controls while leveraging their existing ISMS foundation. In short, ISO 27001 focuses on securing data and systems, while ISO 42001 extends those principles to the realm of AI ethics, bias, and algorithmic risks – together they ensure AI is deployed securely and responsibly.
ISO/IEC 27701 (Privacy Information Management)
ISO 27701 is an extension of 27001 that addresses privacy protection and compliance with data privacy regulations (like GDPR). When an AI system processes personally identifiable information (PII), integrating ISO 27701 into the AI management system is crucial to cover privacy objectives and controls. Annex D points out that organizations should incorporate privacy considerations into the AIMS, referencing ISO 27701 for guidance on how to handle personal data within AI processes. In practical terms, this means the AIMS should adopt privacy risk assessments, data handling procedures, and roles like Data Protection Officer or “PII controller/processor” responsibilities in line with ISO 27701. For example, Clause 4 of ISO 42001 (Context of the organization) would include determining the organization’s role as a PII controller or processor if applicable. Controls in ISO 42001 related to communication and incident response should reference privacy breach handling as detailed in ISO 27701.
With integrating a Privacy Information Management System (PIMS) with the AIMS, organizations ensure that any AI solution handling personal data complies with privacy principles such as consent, purpose limitation, and data minimization. The AIMS would then not only manage ethical AI risks but also enforce that AI algorithms do not violate privacy rights – for instance, ensuring datasets are anonymized or that model outputs don’t inadvertently reveal sensitive information. The key similarity is the risk-based approach: both standards require identifying and mitigating risks (security or privacy) to individuals. The difference is in scope – ISO 27701 is about protecting personal data, whereas ISO 42001 is broader, covering ethical and societal risks of AI. Together, they enable AI that is both privacy-preserving and trustworthy. Annex D specifically mentions ISO 27701 as a reference for certain AI control guidelines (like assessing AI’s impact on individuals), indicating that privacy must be “baked into” AI governance for relevant use cases.
ISO 9001 (Quality Management)
ISO 9001 sets out criteria for a quality management system (QMS) focused on meeting customer requirements and continual improvement of process quality. ISO 42001 can be aligned with ISO 9001 to ensure AI systems are developed and operated with quality in mind. In fact, Annex D includes guidance on integrating with ISO 9001 “for quality management within AI development where life and safety may be at stake”. This suggests that industries producing AI-related products (e.g. automotive or medical AI applications) should treat AI system performance and safety as part of their quality objectives.
Similarities between ISO 42001 and ISO 9001 include the emphasis on defining objectives, roles, document control, competency and awareness, and continuous improvement. The difference is that ISO 9001 is product/service-agnostic, whereas ISO 42001 introduces AI-specific quality factors (like data quality, algorithmic accuracy, and absence of bias as part of “quality”). An integrated approach might involve incorporating AI-specific criteria into the organization’s quality policy and procedures. For example, software quality assurance processes (in line with ISO 9001) would be expanded to cover machine learning model validation, testing for bias or drift, etc., as required by the AIMS. Organizations can use ISO 9001’s structure (plan-do-check-act cycle) to continuously improve AI systems – e.g. using nonconformity and corrective action processes on ethical nonconformities or AI errors.
Through aligning AIMS with the QMS, AI development becomes subject to the same rigor of review, testing, and customer feedback loops as any other product, ensuring reliable and safe AI outcomes. Annex D’s guidance effectively encourages industries like aviation, automotive, healthcare (where quality is directly tied to safety) to merge AI governance with their quality systems to avoid any gaps in oversight.
Sector-Specific Standards (e.g. ISO 22000 for Food Safety, ISO 13485 for Medical Devices)
In addition to generic standards above, Annex D recognizes the need to integrate the AIMS with industry-specific management systems to address domain-specific risks.
For instance, ISO 22000 (Food Safety Management System) is critical in the food industry to control hazards and ensure safe food production. If an organization uses AI in food production or supply (say, an AI that monitors quality on a production line or manages supply chain logistics), the AI’s functioning should be incorporated into the HACCP-based controls of ISO 22000. This might mean validating that an AI vision system correctly detects contaminants (as a critical control point measure) and having contingency procedures if the AI fails. An integrated AIMS with ISO 22000 would ensure the AI systems do not introduce new food safety risks – e.g. by requiring periodic review of the AI’s decisions by food safety experts, or ensuring traceability of AI recommendations affecting food quality.
Similarly, ISO 13485 (Quality Management for Medical Devices) is mandatory for medical device manufacturers to ensure safety and regulatory compliance of devices. These days, many medical devices incorporate AI (for example, diagnostic algorithms in imaging equipment or AI-driven patient monitoring tools). Integrating ISO 42001 with ISO 13485 means that the governance of the AI components (data training, algorithm validation, bias monitoring) becomes part of the medical device quality system. Key similarities are that both ISO 13485 and ISO 42001 demand risk management – ISO 13485 requires risk management for patient safety, and ISO 42001 requires risk management for AI trustworthiness. Thus, an organization making an AI-based medical device would perform a unified risk assessment that covers traditional product risks (e.g. electrical safety, biocompatibility) and AI-specific risks (e.g. incorrect outputs or bias), aligning with both standards’ requirements.
Annex D highlights that applying the AI management system in such regulated sectors should enhance overall compliance and effectiveness.
In practice, this alignment helps avoid conflicts – for example, ensuring that the AI’s behavior does not violate any medical device regulatory requirements. It also ensures that domain-specific best practices (like validation protocols from healthcare, or safety practices from industrial automation standards) are applied to AI. Ultimately, by mapping AI controls to existing sector standards, organizations can maintain consistency: the AI management system becomes an extension of their current management system, speaking the language of that industry.
This is how ISO 42001 remains flexible yet comprehensive – it explicitly encourages organizations to “plug in” the AIMS into their established compliance framework, whether that is for food safety, medical device quality, automotive safety (e.g. ISO 26262) or any other field.
Primary Similarities and Differences
All these management system standards share a common emphasis on governance, documented processes, risk-based thinking, and continuous improvement. This makes it feasible to integrate them – for example, ISO 42001 adopts the harmonized structure to enhance alignment with standards related to quality, safety, security, and privacy.
Policies and controls can often be shared or cross-referenced. However, the content focus differs: ISO 27001 is about safeguarding information, ISO 27701 about handling personal data, ISO 9001 about meeting customer and regulatory requirements for quality, and ISO 42001 about ensuring AI is trustworthy (safe, fair, transparent, etc.). When aligning them, organizations must map where objectives intersect and where new controls are needed. A simple view is that AI management systems will inherit many controls from existing regimes (especially in security and quality), and add new controls addressing AI ethics, bias, algorithmic transparency, and AI-specific safety concerns. Aligning objectives and controls across these standards, organizations create an “integrated management system” that covers multiple facets – for example, a single audit could check compliance with ISO 27001, 27701, 9001, and 42001 together, since the systems are interwoven.
Annex D guides organizations to leverage what they already have: if you have an ISMS, a QMS, or sector certifications, build your AI management on top of those. Doing so, AI governance becomes another thread in the fabric of enterprise governance, rather than a separate silo. This integrated approach is efficient (minimizes duplicate documentation and audits) and effective (ensures AI risks are not overlooked in the broader corporate risk context). Companies that have successfully aligned ISO 42001 with, say, ISO 27001 have reported reduced administrative workload and more coherent policies. Integrated teams can respond to incidents in a coordinated way – e.g. a data breach in an AI system would trigger both AI risk controls and infosec controls in unison.
These synergies make a compelling case for organizations to treat AIMS as an extension of their existing management systems rather than something entirely new.
Sector-Specific Application of AI Management Systems
AI is being deployed across virtually every sector, but the risks, regulatory requirements, and ethical concerns can vary significantly by industry.
ISO 42001’s Annex D acknowledges this by providing industry-specific considerations, and organizations are indeed configuring their AI management systems to their sector context.
The following is an in-depth look at how AIMS principles apply in different sectors – including healthcare, defense, transport, finance, employment, and energy – along with examples and (where possible) real implementations:
Healthcare Sector
In healthcare, AI systems are used for applications like diagnostic image analysis, predictive analytics for patient outcomes, robot-assisted surgery, and administrative decision-making. The stakes are extremely high – patient safety, privacy, and ethics are paramount. An AI management system in healthcare must therefore ensure that AI tools are safe, effective, and aligned with medical ethics and regulations. Annex D specifically notes healthcare as a domain where the AI management system is applicableand provides guidance on integrating with standards like those for medical devices.
Example & Implementation
A notable real-world example is Emirates Health Services (EHS) in the UAE, which became one of the first organizations to be assessed against ISO 42001. EHS applied an AIMS to govern AI deployment in areas like medical imaging diagnostics. This helped ensure their AI systems are used responsibly to enhance patient care while prioritizing ethics, transparency, and patient safety. By aligning with ISO 42001, EHS could demonstrably show that their AI imaging tools were being managed under a rigorous framework – covering everything from data quality and bias checks to accountability for AI decisions. According to EHS leadership, implementing the AI management standard was “helpful in our journey of deploying AI in different areas of medical imaging”, reinforcing their commitment to safe and ethical AI-driven healthcare solutions. This case illustrates how an AIMS can be integrated into a healthcare organization’s operations: it likely involved setting an AI policy aligned with healthcare ethics (e.g. adhering to the principle of “do no harm”), conducting risk assessments for AI errors or biases that could affect diagnoses, and ensuring compliance with health regulations like HIPAA (for privacy) or FDA guidelines for AI/ML-based medical devices.
Significant considerations in healthcare
Data privacy is crucial (patient health records are highly sensitive), so the AIMS must include strict controls on data use, in line with standards like ISO 27701 and health-specific privacy laws. Another consideration is algorithm bias – for example, if an AI system for diagnosing skin cancer was trained mostly on lighter skin tones, it might under-diagnose patients with darker skin.
A robust AI management system would catch this risk (through bias testing processes) and require mitigations (such as retraining the model on a more diverse dataset). Additionally, AI outcomes in healthcare often need to be explainable due to liability and trust reasons – doctors need to understand AI recommendations. The AIMS therefore encourages explainability and human oversight controls (as referenced in ISO 42001’s Annex B and other AI standards) to ensure clinicians can interpret and, if necessary, override AI decisions.
Healthcare organizations also face regulatory oversight (e.g. the EU Medical Device Regulation now covers certain AI software). An AIMS helps by systematically addressing these compliance requirements. For instance, if an AI is considered a medical device, ISO 42001 can integrate with ISO 13485 as discussed, so that regulatory documentation and risk management processes are unified. Hospitals and medical AI developers that implement ISO 42001 are essentially building a culture of AI safety akin to their culture of patient safety – embedding ethical review boards for AI, validating AI like they validate new medical treatments, and continuously monitoring AI performance (for example, monitoring an AI diagnostic tool’s accuracy drift over time). This sector stands to benefit greatly from AIMS because trust is a currency in healthcare: practitioners and patients must trust the AI.
Following a certified management system, a healthcare provider can demonstrate that trust is well-placed, potentially improving adoption of beneficial AI technologies.
Defense and Security Sector
The defense sector’s use of AI ranges from intelligence analysis, surveillance systems, cybersecurity defense, logistics optimization, to experimental uses like autonomous vehicles or decision support in combat scenarios. AI in defense is often mission-critical and can have life-or-death consequences, raising unique ethical and operational challenges. Annex D explicitly lists defense as a sector addressed by the standard, reflecting the need for guidance in this area.
An AI management system in defense must contend with issues of safety, reliability, and ethical constraints (like adherence to international humanitarian law) when deploying AI. For example, if AI is used to control or assist weapons systems, robust governance is needed to ensure human oversight and prevent unintended engagements. Many defense organizations (like the U.S. Department of Defense) have already published AI ethical principles – such as being responsible, equitable, traceable, reliable, and governable – which align closely with ISO 42001’s trustworthiness attributes. The AIMS provides a structured way to implement those principles through concrete processes and controls.
Significant considerations in defense
Reliability and testing are vital. Defense AI systems must be tested in a wide range of scenarios (including adversarial conditions) to ensure they don’t fail unexpectedly. The AIMS would enforce comprehensive risk assessment for AI failures – e.g. what if an AI misidentifies a target? – and require controls like fail-safes or human confirmation for high-risk decisions. Security is another major factor: defense AIs could be targets of cyber-attacks (poisoning or spoofing attempts by adversaries). Integrating ISO 42001 with ISO 27001/ISO 27036 (supplier security) is vital to secure the AI supply chain. Also, bias and ethics have unique flavors here: an AI used in surveillance should not unfairly target certain populations, and use of AI in surveillance or autonomous weapons raises legal and moral issues. An AIMS can ensure there is an ethical review committee or chain-of-command oversight for AI deployments, evaluating them against rules of engagement and laws of war.
While specific case studies in defense may be classified, one can imagine a defense agency using ISO 42001 to govern an AI project for imagery analysis: the AIMS would ensure data training followed proper handling (no violation of privacy of citizens), the model’s accuracy was validated to a high standard, false positives/negatives are within acceptable bounds, and there are clear accountability lines (who takes decisions based on AI output). Another area is military procurement: contractors providing AI systems might be required to have ISO 42001 certification to assure the military that the product was built and tested under a rigorous management system.
Notably, international bodies are looking at frameworks for military AI governance. A standardized AIMS can complement those efforts by providing a common baseline for managing AI risks in defense globally, potentially building trust among allies that AI is being used responsibly. The challenge in defense is balancing secrecy and agility with governance – ISO 42001 gives a flexible framework that can be adapted to secure environments (where data is classified and can’t be freely shared, for instance, the AIMS documentation would be handled in secure channels).
The defense sector requires that AI be ultra-reliable and used within strict ethical confines. An AI management system helps defense organizations methodically address these requirements, reducing the risk of catastrophic failures or unethical outcomes.
As one Carnegie Endowment report noted, a clear governance strategy is needed so militaries “approach these technologies responsibly”– ISO 42001 can be an instrument to achieve that, by embedding responsibility and accountability into the AI development lifecycle in defense.
Transport (Autonomous Vehicles & Transportation Systems)
The transport sector has seen a surge in AI integration: self-driving cars, intelligent traffic management, predictive maintenance for aircraft and trains, ridesharing and logistics optimization, etc. The benefits of AI here are improved safety (by reducing human error), efficiency, and convenience – but the risks can be literally life-threatening if not managed. An AI management system in the transport sector focuses on functional safety, reliability, and compliance with transportation safety standards.
Applications and Challenges
In automotive, AI systems (like autonomous driving software or driver assistance systems) must comply with functional safety standards such as ISO 26262, and increasingly new standards like ISO 21448 (safety of the intended functionality for autonomous driving) or regulations being developed for autonomous vehicles. Integrating ISO 42001 with these means ensuring the organization’s AI development processes include hazard analysis and risk mitigation for scenarios like sensor failures or algorithm misclassification (for example, mistaking a plastic bag for a rock on the road). AIMS would require continuous monitoring of the AI’s performance on the road (Clause 9 – performance evaluation) and processes for model updates with safety validation (Clause 8 – operations). It would also ensure a transparent reporting culture – if the AI has a near-miss or an incident, it’s analyzed and corrected (analogous to how aviation investigates incidents).
Real-world example
The importance of rigorous AI management in transport was highlighted by the fatal crash of an Uber self-driving test vehicle in 2018 – the first pedestrian fatality caused by an autonomous car. Investigators (the NTSB) later found shortcomings in Uber’s safety culture and test practices, sharply criticizing the company for failing to have adequate risk mitigation and oversight in its self-driving program. For instance, the vehicle’s AI had detected the pedestrian but did not properly classify or react in time, and Uber had disabled a built-in emergency braking feature. An effective AIMS could have enforced stricter testing protocols, redundancy, and real-time intervention controls.
Following that incident, many companies paused autonomous vehicle testing to re-evaluate safety. This case underlines that without a management system ensuring comprehensive risk assessment and fail-safes, AI in transport can lead to tragic outcomes. ISO 42001 provides a framework to prevent such scenarios, requiring, for example, scenario-based risk assessments and clear allocation of responsibilities (there should have been clarity on the backup driver’s role and system limits – topics an AIMS would document).
Beyond autonomous cars, consider public transport and aviation: Airlines might use AI for predictive maintenance of engines. If that AI fails to predict a component fatigue, it could lead to failure in flight. Therefore, an AIMS in an airline would integrate with their safety management system (often following ICAO guidelines or ISO 55001 for asset management) to treat AI predictions with the same rigor as any engineering analysis – verifying the AI’s outputs and setting conservative thresholds for action. In rail transport, AI might manage signaling; an error could cause accidents, so it must be governed by strict safety cases.
Significant considerations
Safety and risk management dominate. The AIMS should enforce that any AI controlling physical systems undergo thorough verification & validation. Another consideration is regulatory compliance – e.g. road authorities might require evidence of safety. An ISO 42001 certification could serve as evidence that a company systematically manages AI safety. Also, cybersecurity intersects here because connected vehicles and transport AIs could be hacked (hence integration with ISO 27001 is relevant, as discussed). Human factors are crucial too: in aviation, pilots work with AI autopilots; mismanagement can cause overreliance or confusion. The AIMS should ensure training programs for human operators to understand AI behavior (Clause 7 – competence and awareness).
Transport organizations that excel in AI governance often share best practices such as “operational design domain” definitions (i.e., clearly specifying the conditions under which an AI can operate safely) and robust change management – if the AI’s software is updated, the change goes through approvals like any major change to a safety-critical system. All these align with ISO 42001’s requirements for planning and control of changes.
The transport sector uses AIMS to systematically minimize AI-related safety risks and build public trust. Doing so, they protect lives and encourage innovation – safe AI deployment means fewer setbacks and greater acceptance of technologies like self-driving cars.
As one analysis of the Uber crash put it, such incidents “magnified the importance of collision avoidance systems” and robust oversight (WikiPedia)– exactly what a structured AI management approach seeks to ensure.
Finance Sector
The finance sector was an early adopter of AI for things like algorithmic trading, fraud detection, credit scoring, insurance underwriting, and robo-advisors for investing. Financial firms are attracted by AI’s ability to analyze large datasets and find patterns (for example, detecting fraudulent transactions in real time, or assessing loan risks faster). However, the finance industry faces strict regulations around fairness, transparency, and accountability – and AI can introduce new compliance challenges. An AI management system in finance aims to ensure that AI algorithms are fair, explainable, secure, and compliant with financial laws and regulations.
Risks and concerns
A major concern in finance is bias and discrimination. AI models might unintentionally discriminate against protected groups. For instance, there was a high-profile case where an AI credit assessment algorithm (used for the Apple Card) appeared to offer significantly lower credit limits to women compared to men with similar profiles. Such bias not only is unethical but can violate equal credit opportunity laws. AIMS in a financial institution would enforce processes to detect and correct bias – e.g. requiring bias testing of models during development and periodic audits of decisions for disparate impact. It would also mandate explainability for high-stakes decisions: regulations often require lenders to provide reasons for adverse decisions, so if AI is involved, the bank must ensure the AI’s decision logic can be interpreted (or an alternative explainable model is used). ISO 42001 supports this by emphasizing transparency and the impact of AI on individuals– controls would be put in place to document how an AI makes decisions and to communicate relevant information to customers.
Another consideration is fraud and security. Banks already comply with ISO 27001 for information security; integrating AIMS means treating models and AI data as assets that need protection. For example, if an AI model that detects money laundering is tampered with, criminals could exploit that. The AIMS would include controls for model integrity (monitoring for data poisoning or model drift that might reduce accuracy).
Governance in finance
Financial services firms typically have strong risk management cultures (e.g. enterprise risk management per Basel II/III, Solvency II, etc.). The AIMS can dovetail with these by adding AI-specific risk criteria into the overall risk register. For instance, a bank’s risk committee would include AI model risk as a category (many banks already have “model risk management” frameworks, guided by regulations like SR 11-7 in the US). ISO 42001 gives a formal structure to model risk management: ensuring every AI model has an owner, is validated before use, monitored during use, and periodically reviewed – much like the expectations of regulators.
A practical example is the use of AI in algorithmic trading: a trading firm would use the AIMS to impose limits on the AI’s actions to prevent erratic behavior (to avoid scenarios like the “Flash Crash”), and to have emergency stop procedures if the AI starts behaving unexpectedly. All these would be documented under Clause 8 (Operation control) and tested under Clause 9 (performance evaluation, e.g. stress-testing the AI under extreme market scenarios).
Real-world status
While specific companies might not publicly announce ISO 42001 adoption yet, many are likely evaluating it. The finance sector’s regulators are encouraging responsible AI – for example, the UK’s FCA and the US CFPB have issued warnings about AI bias. An AIMS could be used by a bank as evidence to regulators that they are managing AI properly. Best practices emerging in finance include establishing internal AI governance committees (with compliance, risk, IT, and business unit participation) to oversee all AI model deployments – effectively an internal audit and approval board for AI. This practice aligns with ISO 42001’s clauses on leadership and organizational roles for AI governance.
In summary
applying an AI management system in finance helps organizations align their AI innovations with the stringent requirements of fairness, accountability, and security that define the financial industry. It reduces risks of non-compliance fines or reputational damage from AI mishaps. And it gives customers and regulators confidence that AI-driven financial services (like deciding a loan or detecting fraud) are being run with the same prudence as traditional processes. As a result, banks can enjoy AI’s efficiency gains without losing trust – a balance that ISO 42001 is explicitly designed to achieve.
Employment & HR Sector
The use of AI in employment contexts (often grouped with “HR tech” or “people analytics”) is growing – examples include AI systems for screening resumes, ranking job candidates, automating interviews (through video analysis), and even AI tools for monitoring employee performance or attrition risk. These applications directly affect individuals’ livelihoods and touch on sensitive issues of fairness, privacy, and transparency.
An AI management system in the employment domain ensures that AI-driven hiring or HR decisions are ethical, unbiased, and compliant with labor and privacy laws.
Challenges
Perhaps the most cited example is Amazon’s experimental recruiting AI that was found to be biased against women – it learned from historical hiring data (mostly male resumes) and started downgrading resumes containing indicators like “women’s” as in “women’s chess club,” etc. Amazon had to scrap this tool upon realizing it “did not like women” and could not guarantee it wouldn’t find other discriminatory proxies. This incident underscores the need for an AIMS to mandate diversity in training data, bias testing, and human oversight in hiring decisions. Under ISO 42001, an organization using AI for recruitment would have controls in place such as: reviewing the AI’s selection criteria for indirect bias, ensuring that AI is only an aid and not the sole decision-maker (to comply with laws that may require human judgment), and providing an avenue for candidates to request reconsideration (an aspect of accountability and transparency).
Privacy is also a major factor – employment-related AI might analyze personal data, including sensitive information. For example, AI might scrape a candidate’s online presence as part of background checks, raising ethical issues. Integrating ISO 27701 here would require consent and clear communication to candidates. If AI is used to monitor employees (e.g. for productivity or security), there are legal boundaries (in some jurisdictions, such monitoring is tightly regulated). The AIMS would ensure any such AI is vetted for necessity and proportionality, and that employees are informed (transparency).
Implementing AIMS in HR
A company could set an AI policy that explicitly forbids the use of attributes like gender, race, or other protected characteristics in algorithms (directly or by proxy), aligning with equal employment opportunity laws. Risk assessment would identify potential for disparate impact. For each AI tool, the HR department might be required to do an impact assessment (similar to a Data Protection Impact Assessment but focused also on fairness and accuracy). Clause 8 of ISO 42001 calls for operational controls – in hiring, that could mean establishing a procedure where any AI-recommended rejection of a candidate is reviewed by a person, or at least periodically auditing the AI’s recommended vs actual hiring outcomes to ensure qualified candidates from any group aren’t consistently overlooked.
Developing best practices
Some organizations have started adopting transparency measures like providing candidates with information on how an AI evaluated them, or allowing appeals – these map to ISO 42001’s focus on transparent and accountable AI. Another practice is bringing in third-party auditors or using bias-detection software on their hiring AIs – effectively a control to ensure the AIMS is working.
Implementing an AIMS, companies in the recruitment space (like LinkedIn with its algorithms, or startups offering AI hiring platforms) can differentiate themselves by showing they follow a responsible AI framework. This not only mitigates legal risks (discrimination lawsuits, which could arise if an AI systematically disadvantaged a group) but also improves their talent acquisition: candidates may feel more comfortable applying if they know AI is used fairly. We’ve seen regulatory movement as well – the city of New York introduced a law that took effect in 2023 requiring audits of AI hiring tools for bias. An ISO 42001 AIMS can help organizations systematically comply with such requirements by building the audit and bias-check processes into their standard operating procedures.
The employment sector uses AIMS to bring accountability to AI-driven HR decisions, treating them with the same seriousness as any other high-impact decision about people. It’s about ensuring that efficiency gained from AI does not come at the cost of fairness or individual rights. A quote from an ACLU piece on AI hiring bias resonates here: “the tool systematically discriminated against women…opening a new frontier for the sector in which regulators are largely unprepared”– ISO 42001 is one way to prepare and put guardrails around this new frontier.
Energy Sector
The energy sector – encompassing electricity generation & distribution, oil and gas, and emerging renewable energy systems – increasingly relies on AI for grid management, demand forecasting, predictive maintenance of infrastructure, optimization of energy production, and even trading energy on markets. The concept of the “smart grid” is heavily driven by AI that can balance loads and integrate renewable sources.
The AI management challenges in energy revolve around safety, reliability, and security of critical infrastructure. Mistakes or attacks involving AI in this sector could lead to power outages or even physical hazards.
Applications and Risks
AI is used to predict equipment failures in power plants or transformers (preventing outages), to automatically reroute power during peak loads, and to manage the variability of renewables by forecasting weather and adjusting storage. While these improve efficiency and can reduce blackout risks, an errant AI decision or a malicious manipulation of AI could have widespread impact. For example, if an AI system mis-forecasts demand or malfunctions in distribution logic, parts of the grid could be left without power unexpectedly. The energy sector has seen incidents of large-scale disruptions (not necessarily caused by AI yet, but the potential exists). One analysis noted that “hallucinations or similar technical glitches could impact energy availability, leaving some businesses and homes without sufficient power”. This highlights the need for thorough validation of AI decisions in the grid context – an AIMS would require simulations and fallback plans if the AI’s output seems aberrant.
Another issue is cybersecurity: Energy infrastructure is a prime target for cyber attacks (as seen in the 2021 Colonial Pipeline ransomware incident or attacks on national grids). AI systems might themselves be targets (for instance, hackers could try to feed false data to an AI that controls grid switching, causing it to misallocate power). Integrating the AIMS with cybersecurity (ISO 27001/IEC 62443 for industrial control systems security) is critical. The AIMS might incorporate controls like data integrity checks for sensor inputs to AI, anomaly detection to flag if the AI recommendations deviate drastically (possibly indicating bad data or a breach), etc. If the AI makes decisions, there must be an override mechanism – e.g. grid operators can manually take control if needed (this aligns with the principle of human-in-the-loop for high-risk AI).
Safety and compliance
The energy sector is highly regulated for safety (consider nuclear power plant operations, which involve rigorous risk management like probabilistic risk assessments). If AI is used in such high-stakes environments (e.g. AI helping to control a nuclear reactor cooling system or directing emergency shutdowns), an AIMS would integrate with the nuclear safety management system, ensuring the AI goes through verification similar to any other safety-critical component. This might include meeting standards like IEC 61508 (functional safety) or country-specific nuclear software guidelines. The AIMS ensures that AI does not override safety margins set by engineers. It would demand extensive testing of AI under abnormal conditions (what if sensors fail or give weird readings? Does the AI gracefully handle it?).
Operational efficiency vs vulnerability
Energy companies also use AI for market trading (buying/selling energy or fuel). There, the risk might be financial or market manipulation if AI behaves in unforeseen ways. The AIMS would incorporate checks to prevent an AI agent from, say, engaging in manipulative strategies or to throttle its activity if it starts causing market anomalies (somewhat analogous to circuit breakers in stock exchanges).
Real-world adoption
Utilities and grid operators are exploring frameworks for AI governance. A possible example: some European grid operators have trialed AI for balancing electricity and had to ensure it met the EU’s network codes and reliability standards. An anecdotal scenario: an AI predicts there’s enough power, but it’s wrong and causes a blackout – the utility would be accountable. To avoid this, they’d only gradually give AI autonomy, under oversight mandated by an AIMS. Interestingly, the energy sector has consortiums and standards emerging for “AI in energy” governance. An ISO 42001 AIMS could give a common approach to evaluate AI solutions from vendors (much like how the sector uses ISO 27019 for SCADA security, etc., they could require ISO 42001 compliance for AI controlling critical functions).
In sum, in the energy sector AI management systems focus on ensuring stability and safety of supply. They apply rigorous risk management to AI algorithms that control physical systems, very similarly to how engineering processes manage any new technology introduction. And they prepare the organization for incidents: just as disaster recovery plans exist for storms and equipment failures, the AIMS would ensure there are contingency plans if the AI system fails or does something unexpected. By doing so, energy companies can harness AI for efficiency (predictive maintenance reducing downtime, smarter grid reducing waste) while maintaining the resilience of the grid.
This balance is crucial; as one RAND report title suggests, there is both “promise and peril of AI in the power grid”– a management system helps maximize the promise and control the peril.
Other Sectors
While the question highlights six sectors, it’s worth noting that ISO 42001’s AIMS is applicable to virtually all sectors where AI is making inroads.
For example, in education, AI is used for student performance analytics and even grading; an AIMS would ensure fairness and data privacy for students.
In manufacturing, AI systems on the factory floor need to be safe for workers and reliable – integration with ISO 45001 (occupational health & safety) could be considered.
In the public sector/government services, AI may be used for things like welfare decisions or policing, which raise serious civil liberties questions – an AIMS in a government agency would introduce accountability and bias monitoring to these use cases, aligning with legal and ethical standards.
Annex D essentially serves as a reminder to all sectors: no matter your domain, if you use AI, adopt a management system approach. The specific controls and emphases will differ, but the baseline of governance, risk management, and continuous improvement is universal. By following Annex D’s guidance, organizations in any industry can map the generic requirements of ISO 42001 to their sector’s context, ensuring the AIMS addresses the most pertinent risks and obligations in that field. The practical examples and case studies (some included in Annex C of the standard) further illustrate how this mapping can be done in real scenarios, guiding organizations on the path from theory to practice.
Comparative Analysis: AI Management System vs. Other Management Systems
When comparing an AI Management System (as specified in ISO 42001) with other established management systems (like information security, privacy, or quality management systems), we find both parallels in governance approach and distinctive elements due to the nature of AI. This section compares the AIMS with other systems in terms of governance structure, compliance focus, risk management, and implementation, and highlights best practices gleaned from industries that have implemented AIMS successfully.
Governance and Structure
ISO 42001 is built on the same high-level governance structure as ISO’s other management system standards. This means it requires top management commitment, clear policies, defined roles and responsibilities, documented processes, monitoring, and continual improvement – all hallmarks of standards like ISO 9001 or ISO 27001.
Organizations implementing an AIMS will recognize the Plan-Do-Check-Act cycle: they must plan how to control AI risks, implement those controls, monitor outcomes, and act on deviations. This similarity allows AIMS governance to be integrated with corporate governance routines (e.g. an AI risk committee might report into an existing enterprise risk management committee). For example, just as ISO 9001 demands a quality policy from leadership, ISO 42001 demands an AI policy endorsed by leadership, ensuring AI is on the executive agenda. A best practice here (observed in early adopters) is to expand the remit of existing governance bodies: many companies have an IT governance or data governance board – they can evolve this into an “AI governance board” to oversee the AIMS, rather than create an entirely new silo.
However, in scope of governance, AIMS has unique facets. Traditional management systems don’t explicitly tackle issues like algorithmic bias, explainability, or automated decision-making impacts. An AIMS brings these into the governance purview. For instance, ISO 42001 explicitly calls out the need to consider “automatic decision-making, non-transparency, and non-explainability” as special factorsthat leadership and governance processes must address. This is a key difference: whereas a quality management system might focus on product defects or customer satisfaction, an AI management system will focus on things like ethical risk, societal impact, and trustworthiness of AI. Thus, governance in AIMS might involve ethicists or legal experts who wouldn’t typically be on a ISO 9001 quality team. An organization noted that implementing AIMS required a “shift in system development approach – using data and machine learning rather than human-coded logic – which changes how systems are justified and controlled”. In governance terms, this means oversight committees need to account for the fact that AI can evolve (learn) and its behavior might not be fully understood by its creators, necessitating continuous oversight.
Compliance and Regulatory Alignment
Each management system standard helps with compliance in its domain: ISO 27001 with data protection laws, ISO 9001 with product regulations and customer requirements, etc. ISO 42001 is similarly geared to help comply with emerging AI regulations (like the EU AI Act or industry-specific AI guidelines). What sets AIMS apart is that the regulatory landscape for AI is new and still evolving, and it spans multiple domains (ethical AI, data privacy, consumer protection, etc.). AIMS provides a proactive framework to manage those obligations collectively. For example, an AIMS can ensure that an organization’s use of AI in credit scoring complies not only with data privacy laws (via integration with PIMS) but also with anti-discrimination laws in lending – by having controls to prevent biased AI outcomes. Other management systems rarely had to explicitly incorporate ethical principles beyond legal compliance, but AIMS does (the EU AI Act, for instance, mandates risk management and data governance for AI, which align with ISO 42001 clauses).
A comparative observation: Many companies already have multiple certifications (say ISO 9001 and ISO 27001). Leading organizations have found that adding ISO 42001 and integrating it yields synergies in compliance.
Best practice example: A tech manufacturer integrated ISO 9001 (quality), ISO 27001 (security), and ISO 42001 (AI) into a single integrated management system. They reported that this “laid a robust groundwork to attain high performance across multiple disciplines”, simplifying compliance across the board. By mapping controls from each standard to the others, they avoided conflicting processes. For instance, change management for software (a quality concern) was linked with change management for AI models (an AI concern) and change management for IT systems (a security concern). This unified approach ensured that whenever a change was made to an AI system, they automatically considered security implications and quality implications at the same time.
Risk Management
All ISO management systems are risk-driven nowadays. ISO 42001 is no exception – it requires identification of AI-related risks and opportunities. In comparison to others, the types of risks have some differences. For an ISMS (ISO 27001), risks might be data breaches or cyber-attacks; for a QMS (ISO 9001), risks might be product failures or customer dissatisfaction; for an AIMS, risks include things like bias harming users, AI decisions causing safety incidents, privacy invasions, lack of transparency eroding user trust, or even strategic risks like an AI behaving unpredictably. That said, the process of risk assessment is similar: define criteria, assess likelihood and impact, implement controls to mitigate.
One difference is that AI risk management must consider a wider array of stakeholders – not just the organization or direct customers, but possibly society at large (if an AI system has societal impact). ISO 42001 explicitly encourages looking at impacts on individuals or groups of individuals, which might extend beyond what a traditional risk assessment would cover. In practice, organizations might add new categories to their risk registers, such as “ethical risk” or “reputational risk from AI outcomes”. Techniques from other domains (like FMEA – failure modes and effects analysis, common in engineering risk management) can be adapted for AI (indeed ISO 23894 for AI risk management, referenced by ISO 42001, guides on this). AIMS and QMS both require continuous risk review and updating controls as needed – with AI, this is particularly important because AI models can “drift” or new risks can emerge as technology or regulations change. This dynamic aspect of AI risk means AIMS may require more frequent risk assessment cycles or real-time monitoring (whereas some other systems can rely on periodic reviews).
A best practice from industry is the use of cross-functional risk workshops when implementing AIMS: e.g. banks bringing together compliance officers, data scientists, and legal counsel to brainstorm AI failure scenarios. This mirrors how info-security risk assessments bring together IT and business stakeholders. The result is a more comprehensive risk treatment plan. Another best practice is leveraging existing risk controls: one company noted that ISO 42001 and ISO 27001 share many controls, so by “leveraging their common aspects, we can simplify processes and documentation, reducing duplication”. For example, if a control exists in ISO 27001 for third-party due diligence, they extended it to cover AI ethics due diligence for vendors under the AIMS, rather than create a new process.
Implementation and Operational Integration
Implementing ISO 42001 can be compared to implementing any management system, but content-wise, it requires involvement of specialized roles (data scientists, ML engineers) and new procedures (like data governance procedures specific to AI training data). Other management systems often align well with certain departments (ISO 9001 with operations/quality dept, ISO 27001 with IT/security dept). ISO 42001 is inherently multi-disciplinary – it touches IT, R&D, compliance, HR (for training/competence), and even PR (for external communication transparency). Implementers in early adopter companies have cited challenges in getting all these players coordinated – but also note that once in place, it becomes a strength (everyone understands their responsibility in AI governance).
In terms of day-to-day operations, an integrated AIMS can streamline work.
Best practice example: One company implemented integrated training programs for staff, covering AI ethics alongside information security awareness, to build a culture of both security and responsibility. By doing this, they created a “competent workforce that can navigate the complexities of AI governance and compliance effectively”. Employees learned not just how to create secure passwords (typical ISMS training) but also how to avoid bias in AI datasets or how to escalate an AI-related concern – thereby operationalizing the AIMS in daily behavior.
Another comparative point is audit and certification: Audits for ISO 42001 can be integrated with audits for other standards. Some organizations have engaged certification bodies to do joint audits. A benefit noted is that having ISO 42001 certification in addition to, say, ISO 27001, can “enhance stakeholder trust in AI capabilities and provide competitive differentiation”. This mirrors how companies use ISO 9001 certification to signal quality excellence. Early adopters in sectors like healthcare (recall EHS example) and tech are touting their ISO 42001 assessments as a mark of honor, indicating they adhere to global best practices in AI governance.
Key Differences
To crystallize, here are some key differences between AIMS and other management systems:
- Subject Matter: AIMS deals with ethical and technical aspects of AI. Other systems deal with security of information, privacy of data, quality of products, etc. AIMS is broader in that AI can impact all those areas, plus societal and ethical dimensions.
- Adaptability: AI technologies evolve rapidly; AIMS must be more adaptive. The standard itself was written to be tech-neutral but expects organizations to update their controls as AI tech changes. Other standards (like quality) may deal with slower-changing processes. This makes continuous improvement especially critical for AIMS – you might need to update your procedures for AI model validation every year as new best practices emerge.
- External Stakeholder Impact: AIMS explicitly considers impacts on external stakeholders (public, customers, etc.) due to AI decisions. Traditional management systems typically focus on the organization’s own outcomes (with the exception of environmental or OH&S systems which consider public and worker safety – interestingly, AIMS has an analogy to those because it cares about public welfare in AI use).
- Transparency Requirement: AIMS has an implicit requirement for algorithmic transparency and communication, which is not a concept in say ISO 27001 or ISO 9001. Those standards don’t mandate explaining your controls to those affected, whereas responsible AI often requires explaining AI decisions to users or those impacted. Organizations implementing AIMS have had to create communication plans about their AI (Annex D even touches on communicating AI-related information in certain sectors). This is a new operational element introduced by AIMS.
Best Practices from Successful Implementations
- Integrate, Integrate, Integrate: Firms that bolted AIMS onto existing systems without integration struggled with complexity. Successful ones treated it as an extension – one unified policy manual with appendices for AI, one integrated risk assessment covering all domains, and combined audits. As EY observed, “integrating standards like ISO 9001, ISO 27001, and ISO 42001 could be an optimal strategy” to achieve performance across disciplines.
- Leverage Existing Strengths: If a company already excels in quality or security management, they leveraged that strength for AI. For example, a company with a strong Six Sigma quality culture used those same methods (define-measure-analyze-improve-control) for improving AI models’ performance under the AIMS, effectively merging quality control with AI control.
- Leadership Buy-in and Culture: Leading adopters ensured top management championed AI ethics, similar to how safety culture is driven from the top. They updated their corporate values or code of conduct to include responsible AI. This cultural embedding meant that employees at all levels became vigilant for AI-related issues (just as in a factory with a quality culture, any worker might call out a defect, in a company with a responsible AI culture, a developer might flag a potential bias issue for management attention).
- Continuous Learning and Adjustment: Firms treating AIMS not as a checkbox but as a learning journey have done well. For instance, after initial implementation, they conduct lessons-learned reviews after each AI project or incident. One best practice is establishing an “AI ethics review board” internally that meets regularly to review difficult cases – this mirrors how some hospitals have ethics committees. Such practices go beyond the strict requirements but greatly enhance the spirit of the AIMS, ensuring it’s a living system.
Concluding the Comparative Analysis
Industries like tech (software companies) have been among the first to align with AIMS because they already had to deal with rapid technology cycles and public scrutiny over AI (e.g. a social media company using AI for content moderation would use an AIMS to reduce occurrences of AI incorrectly censoring or not catching harmful content, which is both a quality and ethics issue for them). Automotive industry players involved in self-driving tech are also adopting rigorous AI system engineering processes akin to AIMS, often combining them with functional safety standards. In banking, as mentioned, model risk management practices are quite mature and essentially perform many AIMS functions; coupling them with ISO 42001 ensures coverage of ethical risks too, not just financial risk.
The comparative takeaway is that ISO 42001’s AI management system aligns well with the governance, risk, and compliance philosophies of other management systems, but it extends them into new territory – dealing with the opaque, dynamic, and potentially impactful nature of AI.
The best practices often involve taking the time-tested elements of older management systems (like documentation discipline, audit trails, corrective action processes) and applying them to AI, while simultaneously addressing new challenges (like needing interdisciplinary collaboration and focusing on ethical outcomes). Organizations that understand this duality are poised to implement AIMS effectively and derive real value (improved AI performance, reduced incidents, greater trust from users and regulators).
Challenges and Limitations in Adopting AI Management Systems
Implementing an AI Management System across various industries is not without obstacles. In fact, early adopters have reported a range of technical, regulatory, and operational challenges when aligning ISO 42001 with their existing processes. Understanding these challenges is important so organizations can prepare and address them proactively.
Common challenges and limitations include:
Aligning AI Initiatives with Business Objectives
Organizations often struggle to integrate AI governance with rapidly evolving business strategies. AI projects move fast and can change scope with new technological possibilities, making it challenging to keep governance policies up to date. Ensuring that the AI management system’s policies remain relevant to the business goals as both the business and AI technology evolve is a real hurdle. For example, a company might roll out a new AI-based service in response to market demand, but if the AIMS policies weren’t updated, that service might launch without fully compliant AI oversight. Maintaining alignment requires continual dialogue between business leaders and the AIMS team, and flexibility to update AI policies whenever the organization’s objectives or use of AI shifts.
Identifying and Assessing AI Risks
AI risks can be novel, complex, and unpredictable. Unlike traditional operational risks, AI failure modes may not be well understood until they occur (e.g. an AI system might work well in test scenarios but err in a corner case). Organizations find it challenging to foresee all potential negative impacts of an AI, especially as threats can rapidly evolve (consider the emergence of adversarial attacks on AI, or new kinds of bias being discovered). This is compounded by the fact that AI systems often behave as “black boxes,” making it hard to know what could go wrong internally. The limitation here is also methodological: many companies lack established methods to quantify AI risks (how do you measure “reputational risk from an AI bias incident” in a risk register?). So there is a learning curve to develop robust AI risk assessment frameworks. The best antidote is to iterate – start with known risks (data security, bias, etc.), then update the risk assessment as new information comes to light. Organizations may need to invest in research or external expertise to properly identify risks (e.g., consulting academic work on AI ethics to learn what to watch out for).
Managing Documentation and Record-Keeping
A complaint from early implementers is the documentation burden. ISO 42001 expects thorough documentation of AI systems: data sources, model information, testing results, decisions made by AI, review records, etc. For companies used to a rapid DevOps style AI development, this can feel cumbersome. Without a structured approach, the volume of documentation (especially for organizations deploying many AI models) can become overwhelming. It’s a challenge to find efficient ways to document things like changes to a machine learning model or results of bias audits in a way that’s useful and compliant but not overly bureaucratic. Some organizations might not have even documented their AI models before (data scientists may prototype models informally); switching to a disciplined documentation practice is a culture shift. Additionally, because AI can be updated frequently (new data, model tuning), documentation can quickly go out of date unless actively managed. This is an area where automation tools can help – for instance, tools that automatically log experiments and model parameters, or integration of documentation steps into the ML pipeline. Still, until such practices are mature, documentation remains a significant effort.
Ensuring Transparency and Explainability
Many AI models, especially complex ones like deep neural networks, lack inherent transparency. Organizations face the limitation that certain AI algorithms are “black boxes” by nature, which makes demonstrating compliance with ISO 42001’s transparency and explainability requirements difficult. Accountability is hard when even developers can’t fully explain why an AI made a specific decision. This challenge is both technical (developing or using explainable AI techniques, which might not exist or may reduce model performance) and procedural (deciding how much explanation is “enough” for compliance or for users). Companies might encounter cases where they have to replace a high-performing but opaque model with a more transparent but slightly less accurate one, to meet governance or regulatory expectations – a tough trade-off. Moreover, explaining AI decisions to end-users in layperson terms is a challenge of its own (communication barrier). This transparency issue is a moving target as well; as public expectation and laws (like the EU AI Act’s transparency obligations) evolve, organizations must continuously improve in this area. Some also struggle with internal transparency: getting AI teams to document their rationale and making that visible to risk managers or auditors can hit resistance if not part of the culture.
Continuous Monitoring and Oversight
AI systems require ongoing oversight because they can change (learn) over time or their operating environment changes. Ensuring continuous performance monitoring and timely intervention is a major challenge. Many organizations are used to a “deploy and done” approach for software – that doesn’t work for AI. They must set up monitoring for model accuracy, bias, drift, etc., and also keep an eye on external factors (like new ethical concerns or new regulations that might affect an AI’s acceptability). This is operationally challenging: it requires infrastructure for monitoring (telemetry from AI systems), processes for periodic review (e.g. monthly model performance reviews), and people with the right skills to interpret the results. It’s essentially adding a new ongoing task to the organizational to-do list, which needs resourcing. Smaller organizations in particular may find it hard to dedicate staff to continuous AI oversight. Additionally, if issues are found, the organization needs agile processes to update the AI system or its controls (which circles back to change management and documentation challenges).
Integrating with Existing Systems and Culture
If an organization already has several management systems (e.g. a bank with ISO 27001 and rigorous governance), adding AIMS might initially create overlap or confusion – employees might be unsure which policy to follow if there’s any inconsistency. Overcoming the silo mentality is a challenge: AI might have been purely an IT or R&D thing before, and now ISO 42001 forces cross-department collaboration (IT, compliance, HR, etc.). Cultural resistance can occur, e.g., data science teams might resist formal oversight fearing it will slow innovation. Similarly, top management might need convincing to invest in what they see as overhead (especially if the ROI of compliance is not immediately visible). This challenge is more subjective but real – organizations must drive home the importance of AI governance to all stakeholders. Some have addressed this by training and awareness sessions (Clause 7 of ISO 42001 emphasizes training and awareness for AIMS), but it takes time for culture to shift.
Technical Limitations
On a technical front, sometimes the tools to implement certain controls are lacking. For example, monitoring an AI for bias in real-time is not straightforward – you might need to collect outcomes over time and analyze them, which requires statistical expertise and tool support. Similarly, measuring qualities like “trustworthiness” or “explainability” can be nebulous. Without clear metrics or industry consensus, organizations can struggle to set meaningful targets (unlike, say, ISO 9001 where you can set targets for defect rates or delivery times). There’s active research and development in AI assurance techniques; early implementers of AIMS are essentially forging new ground and have to tolerate ambiguity or develop custom methods until standards catch up with practice.
Regulatory Uncertainty
While ISO 42001 is meant to help with compliance, many AI laws are in draft or evolving (the EU AI Act, various national AI policies, etc.). Organizations implementing AIMS might be doing so ahead of regulation, which is good for future-proofing but can be challenging because they are not sure exactly what will be required by law. They may need to anticipate and adjust as laws pass. This is both a challenge and a benefit – challenge because it’s uncertainty, benefit because AIMS gives a framework to handle changes. But practically, companies might design a control one way and then have to tweak it once a law is finalized.
To summarize
Given these challenges, it’s clear that adopting an AI management system is not a trivial task. It’s often more complex than implementing older management systems, because AI itself is complex and somewhat uncharted territory. The limitations also mean organizations might not see immediate perfection – e.g. bias might not be eliminated overnight by having an AIMS, but the AIMS provides a path to systematically reduce it. Many challenges can be mitigated by strong leadership support and sufficient resources (time, budget, tools). The organizations that succeeded tended to acknowledge these roadblocks upfront and address them (for example, one company conducted a pilot project on one AI system first, to learn how to document and monitor, then scaled those lessons to all AI projects).
The main obstacles in cross-industry AIMS adoption include keeping AI governance aligned with fast-moving business and tech changes, developing robust AI risk identification methods, handling the increase in documentation and oversight work, opening up “black box” AI for accountability, maintaining continuous vigilance on AI systems, and overcoming integration and cultural hurdles.
None of these are insurmountable – but they require careful planning, possibly new investments (in tools or expertise), and a phased approach to implementation. By being aware of these challenges, organizations can proactively devise strategies (some of which are covered in the next section on recommendations) to ensure their AI management system is sustainable and effective.
Recommendations and Best Practices for Integrating AI Management with Existing Frameworks
For organizations looking to implement ISO/IEC 42001 and integrate an AI management system into their existing governance framework, here are recommendations and best practices drawn from industry insights and the structure of the standard. These steps can help ensure responsible AI development and use in line with both ISO 42001 and other organizational objectives:
1. Start with a Gap Analysis and Strategic Plan
Begin by assessing your current AI practices against ISO 42001 requirements. This involves reviewing existing policies, processes, and controls related to AI (if any) and identifying gaps or weaknesses. Many organizations find it useful to perform an internal audit or hire an expert to benchmark their status. For example, check if you have an AI policy; if not, that’s a gap. Check if AI risks are documented in a risk register; if not, plan to include them. From this analysis, develop a comprehensive implementation plan with clear milestones. The plan should outline which business units or AI systems to tackle first, resources needed, and a timeline. Prioritize high-risk AI applications for early inclusion in the AIMS. This phased approach prevents overwhelm and builds momentum as you can demonstrate quick wins (e.g., establishing an AI risk assessment procedure within one department before scaling it).
2. Gain Leadership Support and Define Governance Structure
Ensure senior management is not just passively supportive but actively engaged. ISO 42001 demands leadership commitment (Clause 5), so educate your C-suite or top executives on the importance of AI governance – perhaps by highlighting regulatory trends or risks of AI incidents. Establish a governance body or steering committee for AI if one doesn’t exist. This could be a new committee or an extension of an existing one (like an IT governance committee). Designate an executive sponsor for the AIMS (for instance, a Chief AI Officer or similar role, or assign it under the CIO or COO). This body will be responsible for oversight of AI policy, risk appetite, and resource allocation. Clearly assign roles and responsibilities related to the AIMS: for example, identify process owners for each clause of the standard – someone in charge of risk management, someone for compliance monitoring, etc. If your organization processes personal data with AI, explicitly assign the role of “PII Controller/Processor” per ISO 27701 within the context of AI operations– often this will be the Data Protection Officer working closely with the AI team. Essentially, bake AI governance into the organizational chart so it’s clear who is responsible for what.
3. Integrate with Existing Management Systems and Policies
Leverage the common structure of ISO standards to merge AI governance with current frameworks. Rather than creating entirely separate documentation, integrate AI considerations into existing documents. For instance, update your information security policy to include AI system security controls, and update your quality policy to mention delivering AI that is trustworthy and meets stakeholder expectations. Align AI risk management with enterprise risk management: add AI risks to the ERM framework so they get evaluated alongside strategic, financial, and operational risks. Map ISO 42001 controls to existing controls from ISO 27001, 9001, etc., to find overlaps – many controls (like access control, incident response, supplier management) can be extended to cover AI systems rather than reinvented. For example, if you have a vendor assessment checklist for security, incorporate AI ethics criteria into it for vendors providing AI solutions (in line with Annex D guidance to integrate with sector standards). Harmonize documentation: use one manual or unified set of procedures that address requirements of multiple standards. This not only reduces workload but also ensures consistency. As noted earlier, integrating management systems can “streamline processes and reduce duplication”, making it easier to maintain compliance across all fronts. Organizations should also ensure that other policies (HR, legal, etc.) align with the AI policy – for instance, your code of conduct could be updated to mention ethical AI use, and HR policies could include repercussions for misuse of AI or for data mishandling. This integration sends a strong message that AI governance is part of the organization’s DNA, not an isolated program.
4. Invest in Training and Awareness
People are at the core of any management system’s success. Provide comprehensive training programs to all relevant staff about the AI management system. This should be role-based: executives need awareness of strategic AI risks and commitments, technical teams need detailed training on procedures for data preparation, model validation, etc., and all employees should get a baseline ethics and compliance training for AI (e.g., what is responsible AI, why bias is harmful, how to flag AI issues). Early adopters have run joint training on AI ethics and info-security to build a holistic mindset. Consider certifying key personnel in ISO 42001 (there are courses and certifications for AIMS implementers and auditors) – this builds internal expertise. Also, conduct drills or workshops, for example, a simulated AI incident (like an AI error causing a major issue) to walk through response processes – similar to how companies do fire drills or security incident drills. Another best practice is to create an internal community of practice for AI developers where governance is a regular topic – sharing lessons and techniques for bias mitigation, for example. By making AI governance part of professional development, you ensure staff competency (Clause 7 requirements on competence and awareness) and get buy-in.
5. Embed AI Risk Management and Compliance Checks into the AI Lifecycle
Make risk management a continuous process, not a one-time activity. Integrate risk and impact assessments at key stages of AI system development and deployment. For instance, require an AI Ethics Impact Assessment or similar review before any new AI system is deployed (this can parallel a Data Privacy Impact Assessment if personal data is involved). Use checklists derived from ISO 42001 Annex B and other guidelines: e.g., have you considered potential bias? Have you considered security? What is the potential impact on individuals? – similar to how safety-critical industries have pre-flight checklists. Additionally, apply continuous monitoring: implement metrics and KPIs for AI performance and trustworthiness (for example, accuracy, incident counts, number of bias complaints, etc.), and review them regularly (Clause 9 – Performance Evaluation). If an AI system is making consequential decisions, set up an auditing process (internal audit function can include AI systems in their scope). Some organizations set thresholds that trigger an alert or review – like if an AI’s accuracy drops below X% or if it starts outputting results outside a certain distribution, someone is notified to investigate. Leverage automation where possible: tools that monitor model drift or fairness can feed into your management system’s monitoring. Incident management is crucial: extend your incident response process to cover AI incidents (for example, if an AI causes a service outage or a scandal due to bias, have a plan ready for response, root cause analysis, stakeholder communication, etc.). Align this with existing business continuity and incident processesso that AI incidents are handled with the same rigor as a cybersecurity incident or a product recall would be. Essentially, treat AI issues not as an anomaly but as part of the operational risk landscape.
6. Nurture a Cross-Functional AI Governance Team or Committee
Assemble a team with representatives from different departments – IT, data science, compliance/legal, risk management, HR, and business units – to oversee and implement the AIMS. This ensures that all perspectives are covered (technical feasibility, legal compliance, ethical implications, business value). This team can be tasked with maintaining the AI risk register, reviewing significant AI decisions, and keeping policies updated with the latest regulations and technologies. For example, privacy officers and security officers should be involved to ensure alignment with 27701 and 27001, respectively, within the AIMS. If the company has an ethics board or similar (some big tech firms do), ensure there is linkage or overlap with the AI governance team. Regular meetings of this group should occur to discuss AI pipeline, upcoming deployments, results of audits, etc. A diverse governance team also helps in spotting issues that a single department might miss – for instance, legal might identify a discrimination law issue that engineers overlooked, or engineers might highlight a technical limitation to an otherwise well-intentioned policy.
7. Incorporate Sector-Specific Requirements and Standards
As recommended by Annex D, tailor your AIMS to your industry. This means actively mapping and incorporating any sectoral guidelines, laws, or standards into your AI controls. For example, a healthcare provider should integrate guidelines from bodies like the FDA (for AI in medical devices) or HIPAA for health data privacy, and perhaps use ISO 13485’s approach to design controls in the AI context. A financial institution should ensure alignment with guidelines from regulators like the Federal Reserve or FINRA on algorithmic trading or credit model governance. Use these external requirements to further refine your AI management system – often, they will dictate certain best practices (like documentation or validation steps) that can be embedded in your procedures. This not only ensures compliance but also demonstrates to regulators that you are pro-active. A tip is to maintain a compliance matrix – list applicable legal/industry requirements and map them to your AIMS controls or policies to ensure coverage. Keep an eye on emerging standards (ISO and others): ISO 42001 references standards like ISO 23894 (AI risk management) and ISO 25059 (AI quality model); incorporating insights from those can strengthen your AIMS. For instance, ISO 25059 can provide quality criteria for AI systems – you could use that to set your quality objectives for AI (Clause 6). Being attuned to sector specifics also helps in staff buy-in – people see that the AIMS is not abstract but solving real issues they face in their domain.
8. Use Technology and Tools to Support AIMS Processes
Given the complexity of AI, manual processes may not be sufficient or efficient. Invest in tools that can assist with AI governance. For example, there are emerging “AI audit” or “AI governance” software platforms that track datasets, models, and experiments, and can generate documentation automatically (ensuring you have those records for ISO 42001). Tools can also help with bias detection, explainability (providing automated explanations for model decisions), and monitoring. Using such tools can embed governance into the AI development environment – so compliance is not an afterthought but built-in. For instance, implement version control for datasets and models, require model cards or datasheets to be filled out (a form of documentation about model intent, performance, limitations), and use pipeline automation to enforce review steps (the pipeline won’t push a model to production unless certain checks are signed off). Additionally, consider tools for managing policies and controls (some GRC – Governance, Risk, Compliance – software can be configured for ISO 42001 to map controls, track compliance status, etc.). By leveraging tech, you also reduce the human workload on repetitive tasks, freeing them to focus on analysis and decision-making.
10. Continuous Improvement and Adaptation
Finally, treat the AI management system as a living program. Schedule regular management reviews (Clause 9) where top management and the AI governance team review the performance of the AIMS: incidents that occurred, audit findings, stakeholder feedback, changing business objectives, new regulations, etc. Use these reviews to identify opportunities to improve the system. Perhaps you find that despite controls, a bias issue slipped through – analyze why and strengthen the process (maybe introduce an additional bias check or external audit). Or if new technology like explainable AI methods become available, update your procedures to use them. Keep an eye on the evolving AI standards landscape and best practices from other organizations; incorporate lessons learned externally. Also, solicit feedback from stakeholders – for instance, user feedback if AI decisions affect customers (did they feel the process was fair and transparent?). This can highlight where the AIMS might need adjustment (like improving communication to users). Maintaining a suggestion program internally (so employees can propose improvements to AI processes) is a good practice too. Remember that ISO 42001 allows flexibility – it’s about meeting objectives, not a rigid checklist. So adapt the implementation as you see what works or doesn’t in your organizational context. Over time, these incremental improvements will mature your AIMS.
11. Build a Culture of Responsible AI
Beyond the formal processes, encourage a corporate culture that supports the AIMS. This means promoting values of ethics, accountability, and quality in AI work. Recognize teams or individuals who identify and address AI risks (positively reinforce the behavior you want). Make responsible AI a part of your brand and internal messaging – when everyone from new hires to the CEO is aware of and proud of the organization’s commitment to trustworthy AI, it creates internal pressure to uphold those standards. Culture will ensure that even when there isn’t a rule for something, people will do the right thing. For example, a developer might catch a potential bias issue and raise it even if a checklist missed it, because they understand it’s part of their responsibility.
Concluding
It’s useful to recall that ISO 42001 is flexible and can be scaled. If you’re a smaller organization or just starting, you might implement a lighter version initially (focus on the key risk controls) and then expand. The best practices above can be right-sized – not every company will need a full dedicated AI committee, but every company should have clear ownership and cross-functional input in some form. The core idea is to embed AI governance into existing structures, rather than bolt-on, and to actively manage AI’s unique risks in a proactive and continuous manner.
Companies that follow these practices are more likely to achieve ISO 42001 certification smoothly and derive real benefits: increased trust from customers and partners, reduced chances of AI failures or legal issues, and improved performance of AI systems due to disciplined monitoring and improvement.
Basically, integrating an AI management system is about building organizational muscle for handling AI responsibly – the recommendations above serve as a workout regimen to develop that muscle effectively. And as AI becomes ever more central to business and society, those who have adopted these best practices early will find themselves ahead of the curve, with robust, trustworthy AI operations that set them apart from competitors.
FAQ
What is Annex D in ISO 42001?
Annex D provides guidance on how an organization’s AI management system (AIMS) applies across various domains (e.g., health, defense, transport, finance). It explains how to integrate AI governance with existing sector-specific and generic management system standards.
Why is Annex D important?
It illustrates the universality of ISO 42001: any organization using AI—regardless of industry—can leverage these guidelines for responsible AI. Annex D helps align AI-specific considerations with broader operational and compliance frameworks.
Which sectors does Annex D specifically mention?
It references health, defense, transport, finance, employment, and energy as examples, but the principles apply to all AI-driven industries.
Does Annex D replace existing management system standards?
No. Annex D highlights how to integrate or align AI management with standards like ISO/IEC 27001 (security), ISO/IEC 27701 (privacy), and ISO 9001 (quality), as well as sector-specific standards like ISO 22000 or ISO 13485.
How does Annex D address cross-industry AI risks?
It specifies the need for a holistic approach: combining AI-specific risks (algorithmic bias, transparency) with existing safety, security, and quality controls. Ensuring comprehensive oversight of AI systems in varied contexts.